Roger Melko
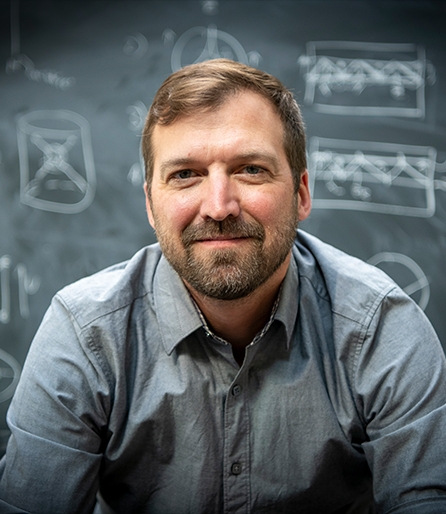
Teaching Affiliations
If you are interested in working with me as a PhD student, please submit an application directly to my department at the University of Waterloo and indicate that you would like to be supervised by me.
Research Interests
My group's interests involve strongly-correlated quantum many-body systems, with a focus on emergent phenomena, novel phases and phase transitions, quantum criticality, and entanglement. We emphasize computational methods as a theoretical technique, in particular the development of state-of-the-art algorithms for the study of strongly-interacting systems. Our work has employed Monte Carlo simulations, density matrix renormalization group, and modern machine learning methods. With these techniques, my group explores low-energy physics in quantum magnets, cold atoms in optical lattices, bosonic fluids, and quantum computers. I am particularly interesting in studying microscopic models that display interesting quantum behavior in the bulk, such as superconducting, spin liquid, topological, or error-correcting phases. We are also interested in broader ideas in computational physics, the development of efficient algorithms for simulating quantum mechanical systems on classical computers, and the relationship of these methods to the fields of machine learning and quantum information science.
Positions Held
- Affiliate Faculty, Vector Institute for Artificial Intelligence, 2017-present
- Scientific Lead, Creative Destruction Labs, 2017-present
- Professor, Department of Physics and Astronomy, University of Waterloo, 2007-present
- Tennessee Wigner Fellow, Oak Ridge National Laboratory, 2005-2007
Awards
- Brockhouse Medal, CAP/DCMMP, 2021
- Discovery Canada Research Chair Western Digital Research Grant, Natural Sciences and Engineering Research Council of Canada (NSERC), 2021
- Herzberg Medal, Canadian Association of Physicists, 2016
- Young Scientist Prize in Computational Physics, International Union of Pure and Applied Physics (IUPAP), 2012
- Early Researcher Award, Province of Ontario, 2010
Recent Publications
- Fitzek, D., Teoh, Y. H., Fung, H. P., Dagnew, G. A., Merali, E., Moss, M. S., . . . Melko, R. G. (2024). RydbergGPT. doi:10.48550/arxiv.2405.21052
- Hibat-Allah, M., Merali, E., Torlai, G., Melko, R. G., & Carrasquilla, J. (2024). Recurrent neural network wave functions for Rydberg atom arrays on kagome lattice. doi:10.48550/arxiv.2405.20384
- Merali, E., De Vlugt, I. J. S., & Melko, R. G. (n.d.). Stochastic series expansion quantum Monte Carlo for Rydberg arrays. SciPost Physics Core, 7(2), 016. doi:10.21468/scipostphyscore.7.2.016
- Luo, X. -Z., Luo, D., & Melko, R. G. (2024). Operator Learning Renormalization Group. doi:10.48550/arxiv.2403.03199
- MacLellan, B., Roztocki, P., Czischek, S., & Melko, R. G. (2024). End-to-end variational quantum sensing. doi:10.48550/arxiv.2403.02394
- King, A. D., Nocera, A., Rams, M. M., Dziarmaga, J., Wiersema, R., Bernoudy, W., . . . Amin, M. H. (2024). Computational supremacy in quantum simulation. doi:10.48550/arxiv.2403.00910
- Moss, M. S., Ebadi, S., Wang, T. T., Semeghini, G., Bohrdt, A., Lukin, M. D., & Melko, R. G. (2024). Enhancing variational Monte Carlo simulations using a programmable quantum simulator. Physical Review A, 109(3), 032410. doi:10.1103/physreva.109.032410
- Torlai, G., & Melko, R. G. (2024). Corner entanglement of a resonating valence bond wavefunction. doi:10.48550/arxiv.2402.17211
- Lin, J., MacLellan, B., Ghanbari, S., Belleville, J., Tran, K., Robichaud, L., . . . Roztocki, P. (2024). GraphiQ: Quantum circuit design for photonic graph states. doi:10.48550/arxiv.2402.09285
- Melko, R. G., & Carrasquilla, J. (2024). Language models for quantum simulation. Nature Computational Science, 4(1), 11-18. doi:10.1038/s43588-023-00578-0
- Hoque, S., Jia, H., Abhishek, A., Fadaie, M., Toledo-Marín, J. Q., Vale, T., . . . Fedorko, W. T. (2023). CaloQVAE : Simulating high-energy particle-calorimeter interactions using hybrid quantum-classical generative models. doi:10.48550/arxiv.2312.03179
- Moss, M. S., Ebadi, S., Wang, T. T., Semeghini, G., Bohrdt, A., Lukin, M. D., & Melko, R. G. (2023). Enhancing variational Monte Carlo using a programmable quantum simulator. doi:10.48550/arxiv.2308.02647
- Hibat-Allah, M., Melko, R. G., & Carrasquilla, J. (2023). Investigating topological order using recurrent neural networks. Physical Review B, 108(7), 075152. doi:10.1103/physrevb.108.075152
- Iouchtchenko, D., Gonthier, J. F., Perdomo-Ortiz, A., & Melko, R. G. (2023). Neural network enhanced measurement efficiency for molecular groundstates. Machine Learning: Science and Technology, 4(1), 015016. doi:10.1088/2632-2153/acb4df
- Merali, E., De Vlugt, I. J. S., & Melko, R. G. (2023). Stochastic Series Expansion Quantum Monte Carlo for Rydberg Arrays. doi:10.21468/scipost.report.6735
- Merali, E., De Vlugt, I. J. S., & Melko, R. G. (2023). Stochastic Series Expansion Quantum Monte Carlo for Rydberg Arrays. doi:10.21468/scipost.report.6713
- Merali, E., De Vlugt, I. J. S., & Melko, R. G. (2023). Stochastic Series Expansion Quantum Monte Carlo for Rydberg Arrays. doi:10.21468/scipost.report.6678
- Bova, F., Goldfarb, A., & Melko, R. G. (2023). Quantum Economic Advantage. Management Science, 69(2), 1116-1126. doi:10.1287/mnsc.2022.4578
- Serwatka, T., Melko, R. G., Burkov, A., & Roy, P. -N. (2023). Quantum Phase Transition in the One-Dimensional Water Chain. Physical Review Letters, 130(2), 026201. doi:10.1103/physrevlett.130.026201
- Wetzel, S. J., Melko, R. G., & Tamblyn, I. (2022). Twin neural network regression is a semi-supervised regression algorithm. Machine Learning: Science and Technology, 3(4), 045007. doi:10.1088/2632-2153/ac9885
- Wetzel, S. J., Ryczko, K., Melko, R. G., & Tamblyn, I. (2022). Twin neural network regression. Applied AI Letters, 3(4). doi:10.1002/ail2.78
- Sehayek, D., & Melko, R. G. (2022). Persistent homology of Z2 gauge theories. Physical Review B, 106(8), 085111. doi:10.1103/physrevb.106.085111
- Hibat-Allah, M., Melko, R. G., & Carrasquilla, J. (2022). Supplementing Recurrent Neural Network Wave Functions with Symmetry and Annealing to Improve Accuracy. doi:10.48550/arxiv.2207.14314
- Timmerman, S. R., Bandic, Z., & Melko, R. (n.d.). Quantum to classical mapping of the two-dimensional toric code in an external field. SciPost Physics Lecture Notes, 057. doi:10.21468/scipostphyslectnotes.57
- Inack, E. M., Morawetz, S., & Melko, R. G. (n.d.). Neural Annealing and Visualization of Autoregressive Neural Networks in the Newman–Moore Model. Condensed Matter, 7(2), 38. doi:10.3390/condmat7020038
- Czischek, S., Moss, M. S., Radzihovsky, M., Merali, E., & Melko, R. G. (2022). Data-enhanced variational Monte Carlo simulations for Rydberg atom arrays. Physical Review B, 105(20), 205108. doi:10.1103/physrevb.105.205108
- Kalinowski, M., Samajdar, R., Melko, R. G., Lukin, M. D., Sachdev, S., & Choi, S. (2022). Bulk and boundary quantum phase transitions in a square Rydberg atom array. Physical Review B, 105(17), 174417. doi:10.1103/physrevb.105.174417
- Inack, E. M., Morawetz, S., & Melko, R. G. (2022). Neural annealing and visualization of autoregressive neural networks in the Newman-Moore model. doi:10.48550/arxiv.2204.11272
- Yon, V., Amirsoleimani, A., Alibart, F., Melko, R. G., Drouin, D., & Beilliard, Y. (n.d.). Exploiting Non-idealities of Resistive Switching Memories for Efficient Machine Learning. Frontiers in Electronics, 3, 825077. doi:10.3389/felec.2022.825077
- Golubeva, A., & Melko, R. G. (2022). Pruning a restricted Boltzmann machine for quantum state reconstruction. Physical Review B, 105(12), 125124. doi:10.1103/physrevb.105.125124
- Czischek, S., Yon, V., Genest, M. -A., Roux, M. -A., Rochette, S., Lemyre, J. C., . . . Melko, R. G. (2022). Miniaturizing neural networks for charge state autotuning in quantum dots. Machine Learning: Science and Technology, 3(1), 015001. doi:10.1088/2632-2153/ac34db
- Ryczko, K., Wetzel, S. J., Melko, R. G., & Tamblyn, I. (2022). Toward Orbital-Free Density Functional Theory with Small Data Sets and Deep Learning. Journal of Chemical Theory and Computation, 18(2), 1122-1128. doi:10.1021/acs.jctc.1c00812
- Timmerman, S. R., Bandic, Z. Z., & Melko, R. G. (2022). Quantum to classical mapping of the two-dimensional toric code in an external field. doi:10.21468/scipost.report.4261
- Czischek, S., Torlai, G., Ray, S., Islam, R., & Melko, R. G. (2021). Simulating a measurement-induced phase transition for trapped-ion circuits. Physical Review A, 104(6), 062405. doi:10.1103/physreva.104.062405
- Bova, F., Goldfarb, A., & Melko, R. G. (2021). Commercial applications of quantum computing. EPJ Quantum Technology, 8(1), 2. doi:10.1140/epjqt/s40507-021-00091-1
- Hibat-Allah, M., Inack, E. M., Wiersema, R., Melko, R. G., & Carrasquilla, J. (2021). Variational neural annealing. Nature Machine Intelligence, 3(11), 952-961. doi:10.1038/s42256-021-00401-3
- Merali, E., De Vlugt, I. J. S., & Melko, R. G. (2021). Stochastic Series Expansion Quantum Monte Carlo for Rydberg Arrays. doi:10.21468/scipost.report.3481
- Merali, E., De Vlugt, I. J. S., & Melko, R. G. (2021). Stochastic Series Expansion Quantum Monte Carlo for Rydberg Arrays. doi:10.21468/scipost.report.3423
- Merali, E., De Vlugt, I. J. S., & Melko, R. G. (2021). Stochastic Series Expansion Quantum Monte Carlo for Rydberg Arrays. doi:10.21468/scipost.report.3360
- Merali, E., De Vlugt, I. J. S., & Melko, R. G. (2021). Stochastic Series Expansion Quantum Monte Carlo for Rydberg Arrays. doi:10.21468/scipost.report.3388
- Merali, E., De Vlugt, I. J. S., & Melko, R. G. (2021). Stochastic Series Expansion Quantum Monte Carlo for Rydberg Arrays. doi:10.48550/arxiv.2107.00766
- Morawetz, S., De Vlugt, I. J. S., Carrasquilla, J., & Melko, R. G. (2021). U(1)-symmetric recurrent neural networks for quantum state reconstruction. Physical Review A, 104(1), 012401. doi:10.1103/physreva.104.012401
- Yevick, D., & Melko, R. (2021). The accuracy of restricted Boltzmann machine models of Ising systems. Computer Physics Communications, 258, 107518. doi:10.1016/j.cpc.2020.107518
Seminars
- Machine Learning Lecture, Machine Learning 2023/24, 2024/04/25, PIRSA:24040053
- Welcome and Opening Remarks, Machine Learning for Quantum Many-Body Systems, 2023/06/12, PIRSA:23060027
- LECTURE: Generative Modelling, Quantum and AI Career Trajectories Mini-Course: Computational Methods and their Applications, 2023/05/10, PIRSA:23050095
- LECTURE: Generative Modelling, Quantum and AI Career Trajectories Mini-Course: Computational Methods and their Applications, 2023/05/09, PIRSA:23050097
- LECTURE: Generative Modelling, Quantum and AI Career Trajectories Mini-Course: Computational Methods and their Applications, 2023/05/08, PIRSA:23050140
- Machine Learning Lecture - 230330, Machine Learning for Many-Body Physics (2022/2023), 2023/03/30, PIRSA:23030037
- Generative models for quantum state reconstruction, University of Waterloo, Computaitonal Mathematics, Waterloo, Canada, 2022/11/30, Video URL
- Generative models for Rydberg atom arrays, NORTIDA, Sweden, 2022/08/31
- Welcome and Opening Remarks, Quantum Criticality: Gauge Fields and Matter, 2022/05/16, PIRSA:22050026
- Machine Learning (2021/2022), Machine Learning (2021/2022), 2022/05/05, PIRSA:22050011
- Quantum simulation with Rydberg atom array, KITP Program: Towards Classically Intractable Quantum Simulations of Physics and Chemistry, Online, 2022/01/18
- Intelligent Machines: Are We Ready?, Public lecture, Bluewater Association for Lifelong Learning, 2022/01/01
- Quantum Simulation and Rydberg Atom Arrays, Boston University, 2022/01/01
- Machine Learning & quantum (many-body) physics, Summer School, Thoulouse, France, 2022/01/01
- Rydberg atom quantum simulation, QHack 2022, Xanadu, 2022/01/01
- Physics, Artificial Intelligence, and Explainability, Mothers and Machine Learning, Vector institute, 2022/01/01
- Computational Methods for Quantum Many-Body Systems: Algorithms, Models and Materials, FOR1807 International Conference 2021, Georg-August-University Göttingen, 2021/09/09